MCM (Mitsubishi Chemical Group)

MCM's Specialty Polymers and Resins European HQ in Newton Aycliffe. Image Credits: MCM.
The company
Mitsubishi Chemical Group (MCM–Mitsubishi Methacrylates) is a leading global manufacturer in the chemical industry, renowned for its diverse array of industrial-grade products.
The Specialty Polymers and Resins European HQ is based at Newton Aycliffe, situated in County Durham in the heart of the North-East of England.
This site was purpose-built for the design and manufacture of acrylic polymers using suspension polymerisation technology. Think of it as a high-tech kitchen in the enormous restaurant of the chemical industry, with a special recipe for creating acrylic polymers. Acrylic polymers are a key ingredient for everything from medical and dental adhesives to various industrial products.
Greg Shaw, a Data Analyst at MCM's Newton Aycliffe plant described the company's core operation as "turning monomers into performance polymers.” Just as a chef carefully combines ingredients to create a perfect dish, this process mixes monomers (the basic building blocks) in a reactor to form polymers, ensuring the product is of the highest quality.
What sets MCM apart in its field is not just its innovative technology but also its strategic use of data. Throughout polymer manufacture, MCM capture a lot of data about the process and product. Greg, who has been with MCM since July 2021, plays a key role in developing reports and analytics to enhance production and commercial efficiency.
The problem
The Newton Aycliffe plant is always striving to increase efficiency and productivity at the site, but the specialty nature of the business means tight product specifications and inevitably some primary batch failures.
Greg explained, "not all of the batches always pass first time" leading to additional reprocessing costs to get the product into specification.
Just how the consistency of ground coffee can affect the flavour of your brew, in the world of polymer production, the uniform distribution of particle sizes is vital to the product's application performance.
While every batch of specialty polymer undergoes strict quality control, the average size of the particles — known as mean particle size distribution (PSD) — can be a frequent reason for primary batch failure.
If the particles are not the right size on average, the batch fails to meet the high standards required for medical and industrial use.
MCM sought to take a proactive approach and leverage its vast array of data from product quality to reactor operation data, to identify the causes of these batch failures.

Simplified high-level overview of process. Image Credits: Canva.
The goal
The motivation behind the initiative was twofold: firstly, to boost the consistency of polymer batches, particularly by reducing particle size discrepancies, and secondly, to facilitate data science skills transfer within the team.
By developing a predictive data model, MCM aspired to achieve the following objectives:
- Understand the underlying causes of batch failures
- To accurately predict particle size and hence grade performance
- To quantify the impact of indirect variables on grade performance
The result
Throughout the project, the focus remained on creating a model that not only provided predictions but also could be a lens through which the production process was viewed, understood, and optimised.
Greg, reflecting on the project, emphasised the enhanced comprehension gained through this work: "We now have an improved understanding of what happens within the reactor due to feature engineering. We have continued to apply the data skills transferred in other projects and Power BI work".
This phase of work highlighted the importance of structured and recorded workflows, guiding current analysis but also establishing a legacy of methodology for MCM.
While the project faced challenges, particularly with the nature and type of available data, it provided MCM with valuable insights.
As Greg noted, "We weren't able to produce a model that was accurate enough to be confident to take into the production process due to the nature of captured data. The process highlighted where we need to improve to employ predictive models in the future.
The project was successful due to the skills transfer by the National Innovation Centre for Data. The project served to validate several long-held assumptions about the manufacturing process, particularly in relation to the part of the processing taking part in the reactor.”
This allowed the team at MCM to save time by eliminating several reasons for batch failures and provided knowledge and skills for future investigation.
Image credit: Canva
Implementation
The project team initiated a series of steps to develop a reliable forecasting tool for polymer production quality:
- Dataset construction: They built a comprehensive dataset with 47 distinct variables. This dataset provided a multifaceted view of the production process, necessary for thorough analysis.
- Adherence to data science best practices: The team followed a structured coding system and meticulously documented each phase. This ensured the project was transparent and the methods used were clear to all involved.
- Data pipelines for consistency: Emphasis was placed on creating reproducible results. Data pipelines were developed to allow for consistent recreation and validation of the predictive model.
- Data foundation for predictive modelling: A solid base was established, which is crucial for developing accurate predictive models.
Technical insight
The collaboration emphasised the importance of addressing data quality and the potential of machine learning models in industrial applications.
The technical strategy included:
- Analytical Tools: Utilising R, including the tidyverse suite, and SQL, the team could navigate and manipulate complex datasets.
- Collaborative Platforms: Git and GitHub were essential for version control and collaboration, allowing the team to track changes and work together seamlessly.
- Visualisation Software: Power BI provided the team with the capabilities to transform data into visual insights, enhancing the decision-making process.

Reactors. Image credits: MCM.
Business impact
Although the primary goal of developing a production-ready predictive model was not met, the project spurred a broader recognition within MCM of the value of data science. It led to improvements in how reactor data is utilised and informed ongoing efforts to enhance data quality across the board.
The rigorous process of creating high-quality datasets has led to more structured data management practices, which can be adopted across the organisation.
The data science project provided an additional advantage; it showcased the potential of data and data science to key stakeholders at MCM, and sparked ideas for future initiatives.
Skills transfer
A significant outcome of the project was the upskilling of MCM staff in data science methodologies, R programming, and machine learning concepts.
Greg’s newfound expertise is now being leveraged in other areas, such as sales forecasting, demonstrating the project's lasting impact beyond its initial scope.
The team also worked with a graduate data analyst throughout this project. He developed skills including:
- Running a data science project,
- Developing machine learning models
- Programming best practices
He has gone on to use these skills in further data science projects at MCM and has spoken at several events about the success of this project.
"It was a pleasure working with Greg on the project with Mitsubishi, guiding him through building data science models in NICD fashion and helping him understand the R language and 'tidyverse' approach. I hope the skills we've transferred are both useful to Greg personally and to MCM as a whole".
Dr Paul Goodman, Innovation Lead, National Innovation Centre for Data
Looking forward
MCM remains committed to integrating data-driven decision-making into its operations.
Greg expressed optimism about applying the skills and insights gained from the collaboration with the National Innovation Centre for Data to future projects, particularly in improving sales forecasting and exploring the potential of generative AI and text data analysis to unlock new operational efficiencies.
Greg commented "The main benefit of the project has been the upskilling in R," noting that "We're going to be using that (knowledge) for the first few months of next year (2024) to see if applying those skills in a sales domain can really add value."
Greg further expressed the unexpected benefit of the skills gained to be applied in new domains within MCM "(The upskilling) enabled us to look into different areas which we wouldn't have been able to do previously."
The successful collaboration and skills transfer have worked synergistically with MCM's digitalisation approach, to ensure that the organisation continues to operate in an increasingly data-centric way, and with a more robust approach to data quality and capture
"Working with NICD on this project was not only fun but gave us some great insight into the potential of our data while also greatly enhancing my data science skills. This has since allowed us to utilise predictive models in other areas of the business".
Greg Shaw, Data Analyst, Mitsubishi Chemical
To find out more about MCM, visit their website.
You can read more of our case studies and sign up to our newsletter to keep up to date with our latest news, events and developments.
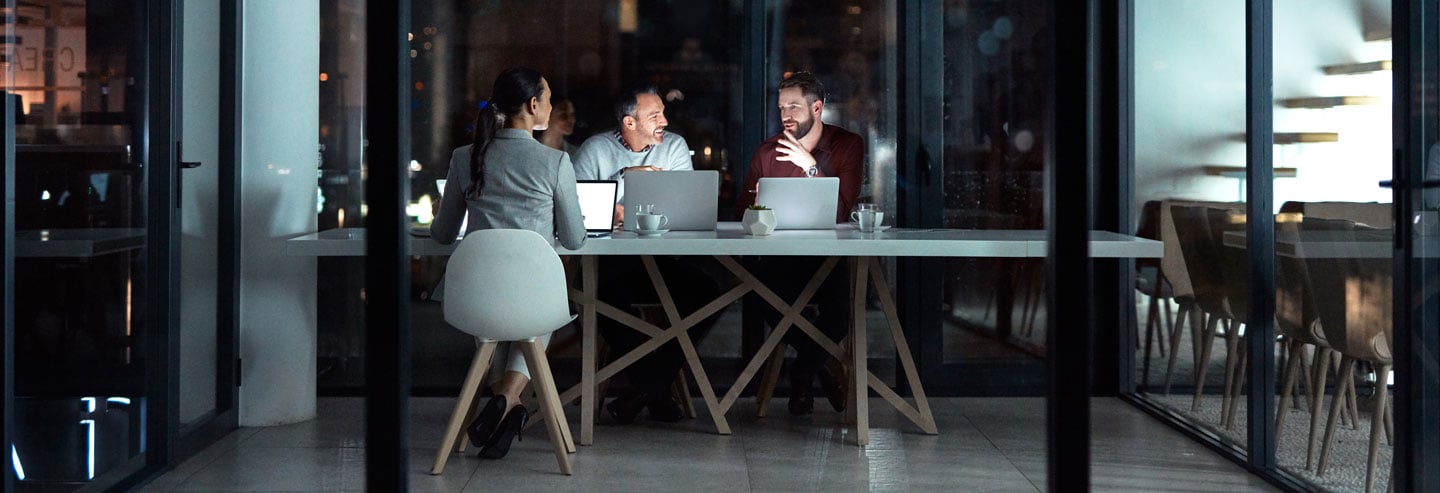
Our Discovery workshop
Our Discovery workshops enable you to explore the potential of your data and understand the benefit you could gain before committing to a full-scale project.